DEVELOPER’s BLOG
技術ブログ
機械学習活用事例|YOLOを使ったドーナツ自動判定
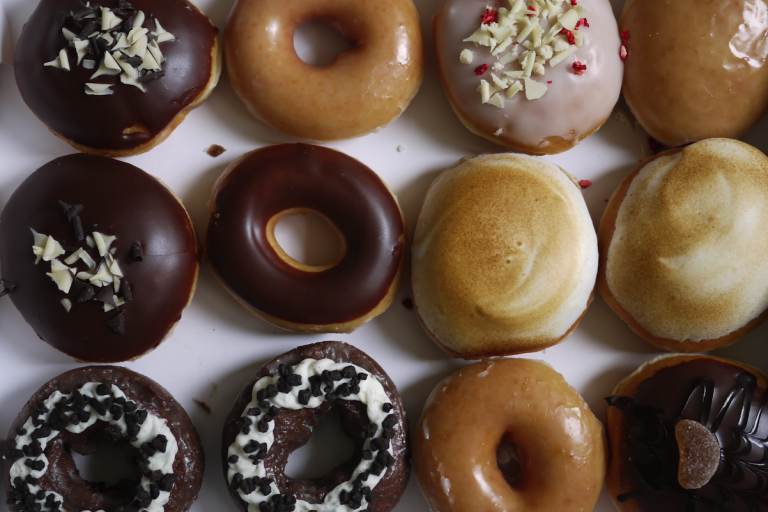
はじめに
このブログは前回、ドーナツの無人レジ化に向け機械学習をどのように用いるかを紹介しました。今回は、その中で出てきたドーナツ検出器の中身について紹介します。
目次
- はじめに
- 検出器を作るために必要なもの
- どのような流れで作るか
- 実際に作る
- まとめ
必要なもの
ドーナツ検出器を作るために、ドーナツの画像データを訓練とテストを用意します。
今回は、「6種類のドーナツを検出し、合計金額を出す」ことが目標として、6種類のドーナツそれぞれの写真を50枚ずつ撮影しました。また、ドーナツが裏返っていても判断できるよう、裏返ったドーナツも同様に撮影しています(訓練データ)。テストデータは複数のドーナツが写る写真を撮りました。このドーナツの中には裏返ったものや写真からきれてしまっているドーナツも含んでいます。
作成手順
ドーナツ検出器をどのような流れで作るかを紹介します。物体検出のためにYOLO3を用います。
大まかな流れとして、このような作業をしています。
- 学習用の画像を整える、画像の水増し
- YOLO3でテスト用データのドーナツ一つ一つを検出する
- 学習モデルを用いてドーナツ名を予測する
- 合計金額などを表示する
1.ではYOLO3を用いて学習用の画像データからドーナツの部分だけを検出します。検出後、画像のアスペクト比(縦横比)を変えずにリサイズをします。画像は一つのドーナツに対して50枚しかないので回転や反転を用いて画像を水増しましょう。一つのドーナツに対して1500枚まで水増ししました。
3.では2.で検出したドーナツ画像から学習モデルを作成します。VGG16を用いて作成しました。
実際に作る
訓練データからドーナツの部分だけ検出する
必要なライブラリをインポートします。
import logging logging.disable(logging.WARNING) import os import glob import numpy as np from keras import backend as K from keras.layers import Input from PIL import Image from yolo import YOLO from PIL import Image from yolo3.utils import letterbox_image from matplotlib import pyplot as plt
keras-yolo3のYOLOクラスを継承してdetect_imageメソッドを修正します。
class CustomYOLO(YOLO): _defaults = { "model_path": 'model_data/yolo.h5', "anchors_path": 'model_data/yolo_anchors.txt', "classes_path": 'model_data/coco_classes.txt', "model_image_size" : (640, 640), "gpu_num" : 1, } def __init__(self, score=0.3, iou=0.45): self.__dict__.update(self._defaults) self.score = score self.iou = iou self.class_names = self._get_class() self.anchors = self._get_anchors() self.sess = K.get_session() self.boxes, self.scores, self.classes = self.generate() def detect_image(self, image): if self.model_image_size != (None, None): assert self.model_image_size[0]%32 == 0, 'Multiples of 32 required' assert self.model_image_size[1]%32 == 0, 'Multiples of 32 required' boxed_image = letterbox_image(image, tuple(reversed(self.model_image_size))) else: new_image_size = (image.width - (image.width % 32), image.height - (image.height % 32)) boxed_image = letterbox_image(image, new_image_size) image_data = np.array(boxed_image, dtype='float32') image_data /= 255. image_data = np.expand_dims(image_data, 0) # Add batch dimension. out_boxes, out_scores, out_classes = self.sess.run( [self.boxes, self.scores, self.classes], feed_dict={ self.yolo_model.input: image_data, self.input_image_shape: [image.size[1], image.size[0]], K.learning_phase(): 0 }) donut_out_boxes = [] for i, c in reversed(list(enumerate(out_classes))): if self.class_names[c] == 'donut': box = out_boxes[i] top, left, bottom, right = box top = max(0, np.floor(top + 0.5).astype('int32') - 100) left = max(0, np.floor(left + 0.5).astype('int32') - 100) bottom = min(image.size[1], np.floor(bottom + 0.5).astype('int32') + 100) right = min(image.size[0], np.floor(right + 0.5).astype('int32') + 100) donut_out_boxes.append([left, top, right, bottom]) return donut_out_boxes
YOLOで訓練画像からドーナツ部分を切り取って画像を保存します。
def crop_donuts(front_or_back): for dir_name in label_dict.keys(): print(dir_name) output_path = '../data/cropped_donut_images/' + front_or_back + '/' + dir_name if not os.path.exists(output_path): os.makedirs(output_path) for file_path in glob.glob('../data/donut_images/' + front_or_back + '/' + dir_name + '/*.jpg'): file_name = file_path[-12:-4] image = Image.open(file_path) out_boxes = yolo.detect_image(image.copy()) if len(out_boxes) == 1: cropped_img = image.crop(out_boxes[0]) cropped_img.save(output_path + '/' + str(file_name) + '.jpg')
訓練画像内にはドーナツが1つしかないはずなので、2つ以上ドーナツを検出した場合は保存せずその画像はスキップします。保存した画像を確認し、検出したがうまく切り取れていない画像はフォルダから削除してください。
YOLOでの検出がうまくいかなかった画像、検出したが切り出しがうまくいかなかった画像を抽出します。
def copy_undetected_images(front_or_back): for dir_name in label_dict.keys(): print(dir_name) output_path = '../data/not_detected_images/' + front_or_back + '/' + dir_name if not os.path.exists(output_path): os.makedirs(output_path) for file_path in glob.glob('../data/donut_images/' + front_or_back + '/' + dir_name + '/*.jpg'): file_name = file_path[-12:-4] path = '../data/cropped_donut_images/' + front_or_back + '/' + dir_name + '/' + file_name + '.jpg' if not os.path.exists(path): image = Image.open(file_path) image.save(output_path + '/' + str(file_name) + '.jpg')
ここで、抽出されたものは、手動でドーナツ部分を切り取りました。
1つ前の関数で抽出した画像をcroppeddonutimagesフォルダ下の適切なフォルダにコピーします。
def copy_images_cropped_by_hands(front_or_back): for dir_name in label_dict.keys(): print(dir_name) output_path = '../data/cropped_donut_images/' + front_or_back + '/' + dir_name if not os.path.exists(output_path): os.makedirs(output_path) for file_path in glob.glob('../data/images_cropped_by_hands/' + front_or_back + '/' + dir_name + '/*.jpg'): file_name = file_path[-12:-4] path = output_path + '/' + file_name + '.jpg' if not os.path.exists(path): image = Image.open(file_path) image.save(path)
ドーナツの画像分類のモデル作成
アスペクト比を変えずに設定した大きさに画像をリサイズします。
def resize(img, base_w, base_h): base_ratio = base_w / base_h img_h, img_w = img.shape[:2] img_ratio = img_w / img_h white_img = np.zeros((base_h, base_w, 3), np.uint8) white_img[:, :] = [220, 220, 220] if img_ratio > base_ratio: h = int(base_w / img_ratio) w = base_w resize_img = cv2.resize(img, (w, h)) else: h = base_h w = int(base_h * img_ratio) resize_img = cv2.resize(img, (w, h)) white_img[int(base_h/2-h/2):int(base_h/2+h/2),int(base_w/2-w/2):int(base_w/2+w/2)] = resize_img resize_img = white_img return resize_img
正解ラベルのエンコード用に辞書を定義します。
label_dict = { 'エンゼルクリーム': 0, 'エンゼルフレンチ': 1, 'オールドファッションハニー': 2, 'ダブルチョコレート': 3, 'チョコファッション': 4, 'ポンデリング': 5, }
データセットを作成します。
front_path = './drive/My Drive/donuts_detection/data/cropped_donut_images/front/' back_path = './drive/My Drive/donuts_detection/data/cropped_donut_images/back/' IMG_SIZE = 256 X = [] y = [] print("== DONUTS FRONT ==") for dir_name in label_dict.keys(): print(dir_name) label = label_dict[str(dir_name)] X_tmp = [] y_tmp = [] for file_path in glob.glob(front_path + dir_name + '/*.jpg'): img = image.load_img(file_path) # img = rotate(img) img = image.img_to_array(img) img = resize(img, IMG_SIZE, IMG_SIZE) X_tmp.append(img) y_tmp.append(label) for x in datagen.flow(np.array(X_tmp), batch_size=1): new_image = x[0] X_tmp.append(new_image) y_tmp.append(label) if len(X_tmp) % 1000 == 0: break X.extend(X_tmp) y.extend(y_tmp) del X_tmp, y_tmp print("== DONUTS BACK ==") for dir_name in label_dict.keys(): print(dir_name) label = label_dict[str(dir_name)] X_tmp = [] y_tmp = [] for file_path in glob.glob(back_path + dir_name + '/*.jpg'): img = image.load_img(file_path) # img = rotate(img) img = image.img_to_array(img) img = resize(img, IMG_SIZE, IMG_SIZE) X_tmp.append(img) y_tmp.append(label) for x in datagen.flow(np.array(X_tmp), batch_size=1): new_image = x[0] X_tmp.append(new_image) y_tmp.append(label) if len(X_tmp) % 1000 == 0: break X.extend(X_tmp) y.extend(y_tmp) X = np.array(X, dtype='float32') X /= 255.0 y = np.array(y) y = np_utils.to_categorical(y, len(label_dict))
データの水増しを行いながらデータセットを作成します。 ドーナツの表面だけ使用するなら、それぞれのラベルにつき1500枚までメモリ不足にならずに水増しできました。表面・裏面とも使用する場合はメモリ容量の関係で各ラベル1000枚まで水増ししました。
モデルを作成します。
def create_model(): base_model=VGG16(weights='imagenet',include_top=False, input_tensor=Input(shape=(IMG_SIZE, IMG_SIZE, 3))) x = base_model.output x = GlobalAveragePooling2D()(x) x = Dense(1024, activation='relu')(x) prediction = Dense(len(label_dict), activation='softmax')(x) model=Model(inputs=base_model.input, outputs=prediction) for layer in base_model.layers: layer.trainable = False model.compile(loss='categorical_crossentropy', optimizer='adam', metrics=['acc']) return model
VGG16の事前学習モデルを利用しました。モデルにVGG16の全結合層は含まず、分類用に独自に全結合層を追加しています。また、VGG16の層は学習しないようにしています。
モデルを学習して保存します。
EPOCHS = 20 BATCH_SIZE = 64 MODEL_PATH = './drive/My Drive/donuts_detection/models/donuts_front_and_back_cropped_model_2.h5' X_train, X_valid, y_train, y_valid = train_test_split(X, y, test_size=0.30) model = create_model() history = model.fit( X_train, y_train, validation_data=[X_valid, y_valid], epochs=EPOCHS, batch_size=BATCH_SIZE ) model.save(MODEL_PATH)
エポック数とバッチサイズは適切なものを選んで下さい。
画像内のドーナツをすべて検出し分類する
必要な辞書を定義します。
label_dict = { 'エンゼルクリーム': 0, 'エンゼルフレンチ': 1, 'オールドファッションハニー': 2, 'ダブルチョコレート': 3, 'チョコファッション': 4, 'ポンデリング': 5, } inverse_dict = dict([(v, k) for k, v in label_dict.items()]) ''' { 商品名: [値段, 熱量(kcal), たんぱく質(g), 脂質(g), 炭水化物(g), 食塩相当量(g)] } ''' menu_dict = { 'エンゼルクリーム': [120, 219, 1.3, 12.0, 26.1, 0.6], 'エンゼルフレンチ': [130, 200, 2.0, 15.5, 12.6, 0.3], 'オールドファッションハニー': [110, 355, 3.4, 18.0, 44.4, 0.8], 'ダブルチョコレート': [130, 265, 3.6, 16.8, 24.3, 0.5], 'チョコファッション': [130, 330, 3.7, 20.8, 31.3, 0.8], 'ポンデリング': [110, 219, 1.3, 12.0, 26.1, 0.6], }
YOLOで取得したバウンディングボックスを元に画像からドーナツ部分をトリミングして分類します。
image = Image.open("../data/donut_images/multiple_front_and_back/IMG_2367.jpg") r_image, out_boxes = yolo.detect_image(image.copy()) plt.figure(figsize=(6, 8)) plt.imshow(r_image) X = [] for box in out_boxes: cropped_img = image.crop(box) cropped_img = np.asarray(cropped_img) cropped_img = resize(cropped_img, IMG_SIZE, IMG_SIZE) X.append(cropped_img) X = np.array(X, dtype='float32') X /= 255.0 preds = model.predict(X) pred_labels = [inverse_dict[pred] for pred in np.argmax(preds, axis=1)] result = np.zeros(6) for label in pred_labels: result += np.array(menu_dict[label]) print() print(pred_labels) print() print(f"値段: {int(result[0])}") print(f"熱量(kcal): {result[1]:.1f}") print(f"たんぱく質(g): {result[2]:.1f}") print(f"脂質(g): {result[3]:.1f}") print(f"炭水化物(g): {result[4]:.1f}") print(f"食塩相当量(g): {result[5]:.1f}") print() image
まとめ
最後にどれだけ正確に判定できているか、分類のパフォーマンスを計算してみましょう。
def extract_labels(row): labels = [] for key in label_dict.keys(): if row[key] == '1': labels.append(key) return labels df = pd.read_csv('../multiple_front_and_back.csv') df['labels'] = df.apply(extract_labels, axis=1) donuts_num = 0 detected_donuts_num = 0 corrected_classified_donuts_num = 0 print('== INCORRECTLY DETECTED ==') for file_path in glob.glob('../data/donut_images/multiple_front_and_back/*.jpg'): file_name = file_path[-12:-4] correct_labels = df[df['file_name'] == file_name]['labels'].values[0] donuts_num += len(correct_labels) image = Image.open(file_path) r_image, out_boxes = yolo.detect_image(image.copy()) X = [] for box in out_boxes: cropped_img = image.crop(box) cropped_img = np.asarray(cropped_img) cropped_img = resize(cropped_img, IMG_SIZE, IMG_SIZE) X.append(cropped_img) X = np.array(X, dtype='float32') X /= 255.0 preds = model.predict(X) pred_labels = [inverse_dict[pred] for pred in np.argmax(preds, axis=1)] if len(pred_labels) != len(correct_labels): print(file_name) for label in pred_labels: if label in correct_labels: corrected_classified_donuts_num += 1 correct_labels.remove(label) detected_donuts_num += len(pred_labels) print('==========================') print() print('The number of all donuts: {:d}'.format(donuts_num)) print('The number of donuts detected by YOLO: {:d}'.format(detected_donuts_num)) print('Percentage of donuts detected by YOLO: {:.2f}'.format(detected_donuts_num / donuts_num)) print('Percentage of donuts classified correctly out of all dontuts: {:.2f}'.format(corrected_classified_donuts_num / donuts_num)) print('Percentage of donuts classified correctly out of detected dontuts: {:.2f}'.format(corrected_classified_donuts_num / detected_donuts_num))
出力結果は以下のようになりました。
== INCORRECTLY DETECTED == IMG_2331 IMG_2344 IMG_2357 IMG_2356 ========================== The number of all donuts: 195 The number of donuts detected by YOLO: 195 Percentage of donuts detected by YOLO: 1.00 Percentage of donuts classified correctly out of all dontuts: 0.96 Percentage of donuts classified correctly out of detected dontuts: 0.96
つまり、ドーナツはYOLOによって100%検出され、すべてのドーナツ(検出されたドーナツ)を96%の割合で正しく分類できています。
このシステムにより、今まで手動で行っていたドーナツのレジ計算を自動で行うことができます。
もちろん、ドーナツだけではなく、お弁当詰めにミスがないかチェックしたり、工場の生産ラインで不良品を弾くこともできます。
Twitter・Facebookで定期的に情報発信しています!
Follow @acceluniverse